Building Ethical Principles In AI
Published on 26 Feb 2022
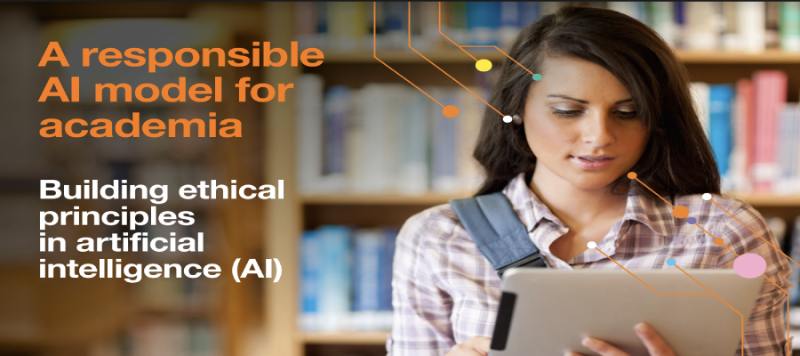
AI is changing the way universities teach and faculty conduct research. However, there are some critical challenges surrounding the usage of AI in education.
These include issues about privacy while gathering significant volumes of data about student competencies. There is also the possibility of bias, both conscious and unconscious, which might have a detrimental influence on outcomes and so on. There are also concerns regarding the quality of data being used; inaccurately measured or fabricated data might yield erroneous findings and create a risky decision-making platform.
See also: VMware Cloud On AWS For Mission-critical Applications
Both supervised and unsupervised learning have drawbacks. The training data set for supervised learning must be authentically representative of the job at hand; otherwise, the AI would demonstrate bias, according to a European Parliament investigation. Unsupervised learning models are promising, but they are computationally difficult, requiring skilled human involvement to modify and evaluate the output.'
Many machine learning models in education are constructed using data produced by humans. These models, however, can only anticipate what they have been trained to predict.
Human biases in training data can readily lead to computational biases if developers do not notice and correct them. AI ethics establish moral standards and governance frameworks to guide AI's development and appropriate application.
Here are five things to think about while implementing AI in academic programs:
- With the frameworks outlined above still in the works, academic institutions must form an AI ethics council to maintain control of AI programs and build trust in AI tools among internal and external stakeholders. The committee can develop an AI roadmap and highlight crucial choices that must be taken. What kind of knowledge is required, for example, and what governance and standards must be established.
- It is critical to building explicit ethical and governance frameworks to guarantee AI solutions comply with regulatory standards such as GDPR.
- According to McKinsey, AI may assist decrease prejudice, but it can also bake in and scale up bias. According to the management consulting organization, the major cause of the problem is underlying data quality rather than algorithms. Preprocessing data is an important step not just to keep as much accuracy as possible, but also to eliminate bias and nonoptimal data.
- The efficacy of AI is dependent on the quality of the data being processed. You must ensure that there are no data leaks in data operations that would pollute the data. The data must also be full, with no discrepancies, or the findings would be erroneous or inadequate.
- Create infrastructure and analytics capabilities from the beginning. A vast amount of data is required to feed AI and construct machine learning algorithms.
Download to read the full whitepaper by Orange Business Services to learn about the importance of building ethical principles in artificial intelligence (AI).