Harness data to reinvent your organization
Published on 08 Oct 2022
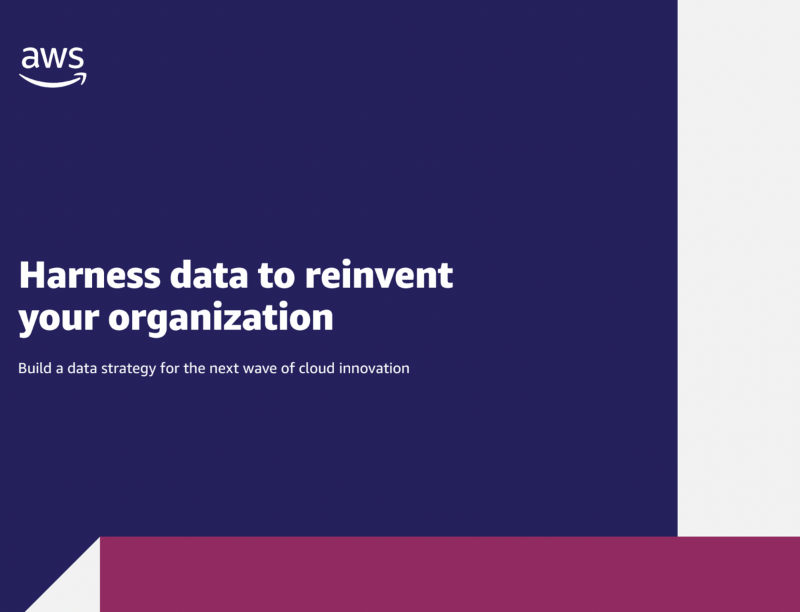
It is difficult for any company to maintain long-term success. Organizations must reinvent themselves regularly to remain relevant. The arrival of the cloud triggered a wave of innovation. Data will now fuel the next round of innovation. Leaders must depend on facts to make sound judgments, see around corners, and take action. Building a data strategy is critical for firms that wish to remain relevant in the present and future.
Leaders who are open to new ideas must be tenacious in their pursuit of the truth. This includes having the tools necessary to pivot in response to opportunities and threats. To accomplish so, you must become data-driven.
Data propel the Road To Reinvention.
Data-driven organizations consider data as an asset, not as the property of particular departments. They build up systems that gather, store, organize, and analyze important data and make it accessible to the people and applications that need it in a secure manner.
These businesses integrate their data into centralized data lakes for easier discovery, governance, and access to gather, store, organize, and act on their information. They also employ machine learning (ML) technology to extract value from their data, such as enhancing operational efficiency, streamlining processes, inventing new products and income streams, and creating better customer experiences.
Moderna and other pharmaceutical firms, for example, are using data and analytics to bring medications and vaccines to market quicker than ever before. BMW and many other firms use data to optimize supply networks and increase production capacity. And, like others in the energy industry, ENGIE utilizes data to develop innovative methods to decrease client costs while reaching ambitious and aspirational environmental targets.
Important concerns and difficulties
The first problem businesses generally encounter is recognizing the sheer number and complexity of the data they manage daily—and the exponential expansion that occurs year after year. Indeed, more data will be generated in the next 36 months than combined in the previous 30 years.
- Old on-premises technologies and older data repositories will not be able to fulfill today's expectations. To handle the vast size and significant development in data quantities that we are seeing today, companies want new data stores that can expand and evolve as business demands change, from gigabytes and terabytes handled now to petabytes and exabytes managed in the future.
- Second, enterprises must be able to readily access and analyze a broader range of data, such as log files, clickstream data, speech, and video. These data types originate from various sources and are kept in silos across numerous data storage. Organizations must break down data silos so that their teams can access and analyze any relevant data, regardless of where it exists, to generate important, fresh insights from all of this data.
- The third main difficulty that firms confront is adjusting to changing client preferences and market dynamics with increased urgency. Organizations must provide their workers with safe access to data and the capacity to do analytics and machine learning on their data in an agile and cost-effective manner to make better and quicker choices. Organizations that rely on old on-premises data infrastructures spend significant time installing hardware and software, configuring the infrastructure for performance and availability, and wasting time on capacity planning to grow their systems. All of this extra work lowers agility and slows decision-making.
- Making machine learning work is the fourth challenge. While machine learning is a disruptive technology that drives innovation, enterprises are having difficulty making substantial progress in expanding machine learning in their operations. According to a Gartner analysis, during the last two years, firms with AI expertise pushed just 53% of their AI proof-of-concept pilots into production. A lack of ML skills, organizational inertia, and a shortage of data to train on are just a few of the obstacles stifling development in this critical sector.
- Finally, in a world where data security, privacy, and compliance rules are becoming more important, companies must be able to identify properly, monitor, and regulate access to particular pieces of data using tried-and-true data governance and security policies. This must be done not just for the data in their particular data silos but also in a comprehensive and consistent manner across all of their data repositories.